TL;DR;
Get the up-to-date data from LINDAS of the currently available values discharge / water level/water temperatures for all BAFU stations.
PREFIX schema: <http://schema.org/>
PREFIX hg: <http://rdf.histograph.io/>
PREFIX la: <https://linked.art/ns/terms/>
prefix ex: <http://example.org/>
# hydro related prefixes
prefix h: <https://environment.ld.admin.ch/foen/hydro/>
prefix hd: <https://environment.ld.admin.ch/foen/hydro/dimension/>
prefix hgs: <https://environment.ld.admin.ch/foen/hydro/station/>
prefix river: <https://environment.ld.admin.ch/foen/hydro/river/observation/>
prefix lake: <https://environment.ld.admin.ch/foen/hydro/lake/observation/>
# geo related prefixes
prefix wkt: <http://www.opengis.net/ont/geosparql#asWKT>
# hydrodata related prefixes (fake) that we use to reference to the historical data.
prefix hydrodata: <https://www.hydrodaten.admin.ch/plots/>
# what we want to get out of the query as "nicely" formatted data
SELECT ?station_id ?station_name ?station_type ?station_type_nice
?latitude ?longitude
?station_iri ?station_data_iri ?station_geometry_iri
(group_concat(?measurement_type_measurement_value;SEPARATOR=";") as ?data)
#?hydrodata_url_temperature ?hydrodata_url_discharge_waterlevel
?query_time
# add the https://hydrodaten.admin.ch url for the data that go back
# 7 days (actually 40 days sometimes, see dev console)
(if(contains(?data,"discharge"),
uri(concat(str(hydrodata:), 'p_q_7days/',
?station_id, '_p_q_7days_de.json')),
"")
as ?hydrodata_url_discharge_waterlevel)
(if(contains(?data,"temp"),
uri(concat(str(hydrodata:), 'temperature_7days/',
?station_id, '_temperature_7days_de.json')),
"")
as ?hydrodata_url_temperature)
where {
# it seems counterintuitive but getting everything and filtering
# is faster than first getting
# the stationlist and go trough their IRI
?station ?prop ?value.
# we only want to keep actual data variables and time
filter( ?prop in (ex:isLiter, hd:measurementTime, hd:waterTemperature, hd:waterLevel, hd:discharge))
# convert the subject station to the id, which is the last thing after the /;
bind(replace(replace(
str(?station), str(river:), "", "i"),
str(lake:), "", "i")
as ?station_id)
# the above is equivalent to this in theory but the above
# uses prefixes so would be easier to change
# in case of changes
#bind(strafter(str(?station), "observation/") as ?station_id2)
# get the kind of station this is (whether lake or river)
# - the hierarchy/ontology here is weird as all are measurement stations
# or observation but the distinction is river/lake first then observation
bind(strbefore(strafter(str(?station), str(h:)), "/") as ?station_type)
# uppercase the first letter -> why is there no capitalize function in SPARQL
bind(concat(ucase(substr(?station_type,1,1)), substr(?station_type,2,strlen(?station_type)-1)) as ?station_type_nice)
# remove some filler words from the data
bind(replace(str(?prop), str(hd:), "", "i") as ?measurement_type)
# convert the actual measurement value to a literal
bind(str(?value) as ?measurement_value)
# introduce the time of the query to (maybe) see if the values are old
bind( str(now()) as ?query_time)
# combine the type of measurement with the value so we
# can group by and not loose information
bind(concat(?measurement_type, ';', ?measurement_value, '') as ?measurement_type_measurement_value)
# start making IRIs so we can check/link back to lindas
# the IRI containing information of the station itself (not linked to river/lake)
bind(IRI(concat(str(hgs:), ?station_id)) as ?station_iri)
# generate the geometry IRI
bind(IRI(concat(str(hgs:), concat(?station_id,"/geometry"))) as ?station_geometry_iri)
# generate the station IRI that holds the data information
bind(IRI(concat(str(h:), ?station_type, "/observation/", ?station_id)) as ?station_data_iri)
# get the information about the location of the station
?station_geometry_iri wkt: ?coordinates.
# also get the name and remove commas to not confuse them with seps
?station_iri schema:name ?stat_name.
bind(replace(?stat_name, ',', ';') as ?station_name)
# simplifiy the geometry to lon/lat in array form
BIND(STRBEFORE(STRAFTER(STR(?coordinates), " "), ")") AS ?latitude)
BIND(STRBEFORE(STRAFTER(STR(?coordinates), "("), " ") AS ?longitude)
}
# group by, basically keep everything of the select here
# except the ?measurement_type_measurement_value which we want to combine to one row
# (ideally we would actually get them as seperate columns again but this seems the best we can do for the moment
group by # the station information
?station_id ?station_type ?station_type_nice ?station_name
?latitude ?longitude
# the IRIs
?station_iri ?station_data_iri ?station_geometry_iri
# validation information
?query_time
This one took quite some time and serves as of now not much purpose other than training SPARQL for me. Essentially, I was asked once to use “the API” for the current water temperatures that are embedded in my RaspberryPI webcams.
Now, initially I actually “webscapped” the JSON data from hydrodata and transferred them to the MCR database as this allowed the concurrent use of in-house data with the BAFU data. Additionally, the urban/rural/water temperature graph of dolueg2 uses exactly the same temperature, meaning it makes sense to keep it in the same database (at least for some of the relevant stations around Basel, the B2091 means BAFU station 2091).
Now going back to “the API” and RDF where data are in triplet form (subject property object) to allow reuse and linking we can get the most recent data of any of the BAFU stations, via e.g.
prefix hd: <https://environment.ld.admin.ch/foen/hydro/dimension/>
SELECT * WHERE {
<https://environment.ld.admin.ch/foen/hydro/river/observation/2091> hd:waterTemperature ?obj .
}
# see also the page: https://environment.ld.admin.ch/foen/hydro/river/observation/2091
or some relevant values for us (more than water temperature)
prefix hd: <https://environment.ld.admin.ch/foen/hydro/dimension/>
prefix obs: <https://environment.ld.admin.ch/foen/hydro/river/observation/>
SELECT * WHERE {
obs:2091 ?prop ?obj .
filter( ?prop in (hd:measurementTime, hd:waterTemperature, hd:waterLevel, hd:discharge))
}

The above is an excerpt of the output from the LINDAS SPARQL endpoint at the time of writing. Notably, we can also get the information for the station (note the added prefix):
prefix station: <https://environment.ld.admin.ch/foen/hydro/station/>
SELECT * WHERE {
station:2091 ?prop ?obj .
}
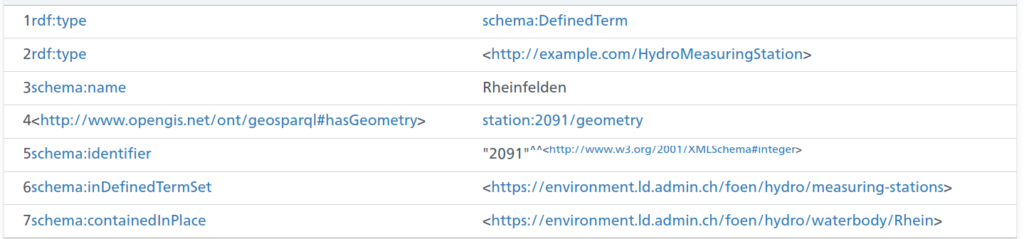
And as you can see, there is also a geometry linked to the station data (shown without query this time):
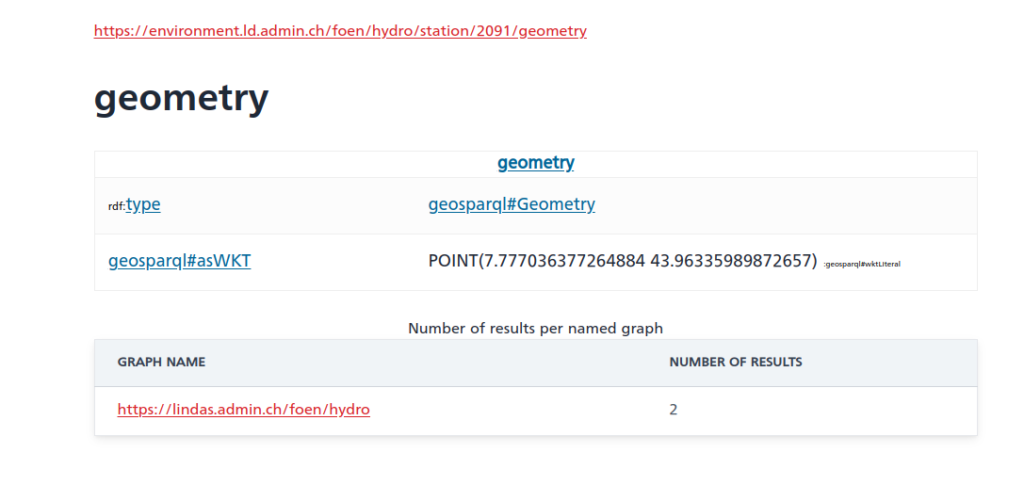
Cool – except for the fact that the southernmost point of Switzerland is located at 45°49’N. And the station in question (id 2091) is in Rheinfelden, i.e. in the North of Switzerland. So something seems off here and I informed the responsible person in the hopes that they’ll fix that soon.
Now, initially, I thought I’d first extract the IDs of the relevant station, then get their properties of interest/values but somehow the query runs rather slow (6+ seconds); probably this could be fixed and if someone wants to try, the following should give a nicely formatted data table (I actually assume that eventually the trigger for measurement time might not be a good choice when more and more datasets would be available on LINDAS):
PREFIX rdf: <http://www.w3.org/1999/02/22-rdf-syntax-ns#>
PREFIX rdfs: <http://www.w3.org/2000/01/rdf-schema#>
PREFIX xsd: <http://www.w3.org/2001/XMLSchema#>
# hydro related prefixes
prefix h: <https://environment.ld.admin.ch/foen/hydro/>
prefix hd: <https://environment.ld.admin.ch/foen/hydro/dimension/>
prefix hydro_geo: <https://environment.ld.admin.ch/foen/hydro/station/>
# geo related prefixes
prefix wkt: <http://www.opengis.net/ont/geosparql#asWKT>
select ?station_iri ?station_id ?station_type ?coordinates ?station_coordinates ?query_time ?measurement_time ?waterTemperature ?discharge ?waterLevel
where {
?station hd:measurementTime ?measurement_time.
optional {?station hd:waterLevel ?waterLevel. }
optional { ?station hd:discharge ?discharge.}
optional { ?station hd:waterTemperature ?waterTemperature.}
# measurement related things
bind(now() as ?query_time)
bind(strbefore(strafter(str(?station), str(h:)), "/") as ?station_type)
bind(strafter(str(?station), "observation/") as ?station_id)
# geo related things
# first find all the relevant subjects, i.e. station property pages
bind(IRI(concat(str(hydro_geo:), concat(?station_id,"/geometry"))) as ?station_geometry_iri)
bind(IRI(concat(str(hydro_geo:), ?station_id)) as ?station_iri)
?station_geometry_iri wkt: ?coordinates.
BIND(strafter(strbefore(str(?coordinates),")"), "POINT(") AS ?station_coordinates)
# can be used for yasgui and nicer representation but slows the query
#bind(xsd:decimal(?station_id) as ?station_id_numeric)
}
#order by desc(?station_id_numeric)

As you can see, it’s important to use the OPTIONAL keyword as not all stations have all variables (water temperature / discharge / level). Additionally, I retrieve the kind of station (lake/river) that is available. The choice of prefix for these is a bit weird for my feeling as the hierarchy seems to be river/lake then station instead of the other way around – which must have a reason that I’m too inexperienced to understand. The rest of the query is cosmetics to get a bit nicer values (keep the geometry as proper type and you can get a map of the observations though).
As seen, this runs rather slow (6+ seconds for around 230 records is too slow on any day) so I started experimenting, arriving ultimately at the code at the top (here shortened to remove geometry and hydrodata links) by first filtering out the relevant dimensions, essentially getting the data first, then cleaning up a bit and concatenating by station id.
PREFIX schema: <http://schema.org/>
PREFIX hg: <http://rdf.histograph.io/>
PREFIX la: <https://linked.art/ns/terms/>
prefix ex: <http://example.org/>
# hydro related prefixes
prefix h: <https://environment.ld.admin.ch/foen/hydro/>
prefix hd: <https://environment.ld.admin.ch/foen/hydro/dimension/>
prefix hgs: <https://environment.ld.admin.ch/foen/hydro/station/>
prefix river: <https://environment.ld.admin.ch/foen/hydro/river/observation/>
prefix lake: <https://environment.ld.admin.ch/foen/hydro/lake/observation/>
# geo related prefixes
prefix wkt: <http://www.opengis.net/ont/geosparql#asWKT>
# what we want to get out of the query as "nicely" formatted data
SELECT ?station_id ?station_name ?station_type ?station_type_nice ?station_iri ?station_data_iri
(group_concat(?measurement_type_measurement_value;SEPARATOR=", ") as ?data)
#?hydrodata_url_temperature ?hydrodata_url_discharge_waterlevel
?query_time
where {
# it seems counterintuitive but getting everything and filtering is faster than first getting
# the stationlist and go trough their IRI
?station ?prop ?value.
# we only want to keep actual data variables and time
filter( ?prop in (hd:measurementTime, hd:waterTemperature, hd:waterLevel, hd:discharge))
# convert the subject station to the id, which is the last thing after the /;
bind(replace(replace(
str(?station), str(river:), "", "i"),
str(lake:), "", "i")
as ?station_id)
# the above is equivalent to this in theory but the above uses prefixes so would be easier to change
# in case of changes
#bind(strafter(str(?station), "observation/") as ?station_id2)
# get the kind of station this is (whether lake or river) - the hierarchy/ontology here is weird as all are measurement stations
# or observation but the distinction is river/lake first then observation
bind(strbefore(strafter(str(?station), str(h:)), "/") as ?station_type)
# uppercase the first letter -> why is there no capitalize function in SPARQL (╯°□°)╯︵ ┻━┻
bind(concat(ucase(substr(?station_type,1,1)), substr(?station_type,2,strlen(?station_type)-1)) as ?station_type_nice)
# remove some filler words from the data
bind(replace(replace(replace(
str(?prop), str(hd:), "", "i"),
"water", "", "i"),
"measurementTime", "measurement_time", "i")
as ?measurement_type)
# convert the actual measurement value to a literal
bind(str(?value) as ?measurement_value)
# introduce the time of the query to (maybe) see if the values are old
bind( str(now()) as ?query_time)
# combine the type of measurement with the value so we can group by and not loose information
bind(concat(?measurement_type, ': ', ?measurement_value, '') as ?measurement_type_measurement_value)
# start making IRIs so we can check/link back to lindas
# the IRI containing information of the station itself (not linked to river/lake)
bind(IRI(concat(str(hgs:), ?station_id)) as ?station_iri)
}
# group by, basically keep everything of the select here
# except the ?measurement_type_measurement_value which we want to combine to one row
# (ideally we would actually get them as seperate columns again but this seems the best we can do for the moment
group by # the station information
?station_id ?station_type ?station_type_nice ?station_name
# the IRIs
?station_iri ?station_data_iri
# validation information
?query_time
This query is the reduced version of the one on top. It runs in around 1 second or less and can now be used for automated queries or other products as described here on LINDAS. The only additions are the added geometry and the URLs for the hydrodata website that give you the historical data to implement a product I have in mind that might come up later. And maybe at some point, we can also get data from the past on LINDAS, making the project I have in mind easier.
Update:
The offset in the geometry seems to be consistently 400’000 m in “hochwert”/latitude based on three stations picked from hydrodata and LINDAS where longitude is almost correct:
b = 2634026 , 1125901 # 2531
a = 7.854351126515431, 42.67878915566025 # 2531
a = wgs84_to_ch1903(*a[::-1], plus=True)[::-1]
print([i - j for i, j in zip(a, b)])
#out: [132.7675012690015, -401020.22037598956]
b = 2627189 , 1267845 # 2091
a = 7.777036377264884, 43.96335989872657 # 2091
a = wgs84_to_ch1903(*a[::-1], plus=True)[::-1]
print([i - j for i, j in zip(a, b)])
#out: [1.471713425591588, -399994.8737920185]
b = 2830800 , 1168706 # 2617
a = 10.265672193049967, 43.036021653211186 # 2617
a = wgs84_to_ch1903(*a[::-1], plus=True)[::-1]
print([i - j for i, j in zip(a, b)])
#out: [48.71478863572702, -399969.5114403835]